A Warped View of Visitor / Customer Analysis?
Many folks look at the world through the lens of the Gaussian Bell Curve when the real model they should be looking at is Pareto Power Law, as elegantly explained by John Hagel here (you really should read that post when you have a chance, another link provided at the end of this post). For the math inclined, as quoted from another source in John’s post:
Gaussian and Paretian distributions differ radically. The main feature of the Gaussian distribution . . . can be entirely characterized by its mean and variance . . . A Paretian distribution does not show a well-behaved mean or variance. A power law, therefore, has no average that can be assumed to represent the typical features of the distribution and no finite standard deviations upon which to base confidence intervals . . .
Yea, like he said. In English, that means when you are “optimizing”, you could be driving towards a suboptimal result if you’re not paying attention to what you should be paying attention to. Witness all the discussion of sample size and standard deviation in web analytics lately - are we talking Bell Curve here, folks? If you walk the Long Tail walk, you should talk the Pareto talk. Here’s a pic from the Hagel blog if you’re not following this idea:
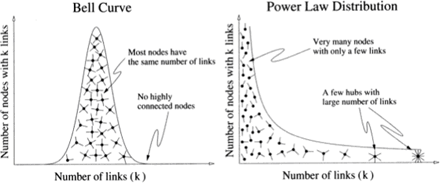
(Image originally by Albert-Laszlo Barabasi, “Linked: The New Science of Networks”)
The bell curve average can kill you. The real results of your tests are masked because you are looking at the wrong outcome variables. Sure, go ahead and segment source; that’s where the idea of “there is no such thing as an average visitor” came from back in 2001, right? So fine; you have the initial segmentation correct, and if you are just optimizing for conversion, you have it right. If you are optimizing for profit, you’re not even close yet. I’ve referred to this situation as the “Reporting versus Analysis” problem; here’s a math-ier view from John’s post, quoting McKelvey and Boisot:
Processing dots is appropriate to what we label the routinizing strategy. Processing patterns, on the other hand, better serves what we call the Pareto-adaptive strategy. Processing dots means processing data, a low-level cognitive activity. By contrast, processing patterns – pattern recognition – is a high-level cognitive activity, one that involves selecting relevant patterns from among myriad possibilities. . .
John offers his own “In other words” on the paragraph above:
In a Paretian world, surface events can become a distraction, diverting attention from the deep structures molding these surface events. Surfaces are extraordinarily complex and rapidly evolving while the deep structures display more simplicity and stability. These deep structures are profoundly historical in nature – they evolve through positive feedback loops and path dependence. Snapshots become misleading and understanding requires a dynamic view of the landscape.
I first saw the effect John describes above at HSN, where the surface (the TV show itself) appeared to be complete chaos but the deep inner structure (the customer) was smooth and completely predictable. Another way to say this is any one ”snapshot” of time seems quite chaotic, but if you watch the whole “movie” over time, you see the stable inner core.
The reverse is also true: as you try to artificially “stabilize” the surface – in HSN’s case, by moving to a rigid or supply-driven programming format rather than a demand or customer-driven format – the core starts to destabilize. Marketing programs stop working as well as they did and the LifeTime Value of the customer erodes. Darwinian, sort of.
What all this means to you: Optimizing for conversion is fine, as far as it goes. But how do you know ”the converted” are the best customers you can get? How do you know you’re not wasting resources optimizing the conversion of worst customers, for example? How do you know that buried in your conversion optimization – no matter how much segmentation you do on the front end – is a Pareto distribution that is skewing the actual results?
Example:
One of my earliest clients was a vintage 1998 dot-com that had a massive business in lead generation. They basically generated e-mail names through various niche interest content sites and then rented those names. Over time, they also started creating their own products and selling to the names they generated. Great business, killer margins. They were a massive buyer of all types of online media.
But then that media started to get much more expensive, and they had to start looking at Campaign ROMI as opposed to just sales, and that is why I was brought in. We were in the middle of a system integration that would provide a “ROMI Dashboard” to the media buyers when the word came down to kill off about 1/3 of the campaigns they were running. I begged them to wait for the data (we’re almost there!) but the “shoot from the hip” culture prevailed.
Pareto had his day. The campaigns killed were the ones with the lowest initial response rate – and as it turns out, these same campaigns also happened to generate about 95% of their most profitable customers. You just had to wait about 3 months after initial conversion to see the profit.
The customer value death spiral (Kevin uses the term file momentum to describe the same idea) ensued and they were never able to recover. Once this kind of best customer value erosion has completed, it is very difficult to survive long enough to rebuild the power of the database. Media costs rose as customer productivity fell and when these two lines crossed, the company collapsed.
This death spiral / file momentum idea is incredibly important in the Marketing Productivity area and somewhat difficult to get your arms around, so it’s worth helping you to visualize it (if you’d like audio with your visualization, try this).
Here we go:
Say that 20% of the customers you acquire are “best customers” and generate 80% of your profits. In profit terms, say this means $200 in net profit per customer over 6 months. Now, let’s say you change your customer acquisition methods and your new “best customers” generate $100 in net profits over 6 months.
The day before you initiate this change in campaigns, you still are generating best customers with a value of $200 over 6 months. So you have about 6 months before the profit effect of these customers disappears. And remember, these folks are responsible for the majority of your profits. Few in number, powerful impact.
So, you start generating customers only worth $100 over 6 months. You don’t even notice anything wrong, since the value plays out over time. Conversions are fine, and sales are fine – because you are still living off the $200 customers while you are bringing in the $100 customers. And the rest of your operations remain the same, so unit sales and so forth seem fine. We’re only talking about a change to a small group of customers, right?
About month 3, sales start to slip, out of nowhere. You’ve entered the wide mouth of the death spiral (cue the audio). You can’t explain it, you haven’t “changed” anything recently. Conversions of those new $100 customers are still coming in just fine, thank you. But after month 6, you start living only on the $100 customers and sales are now 3/4 what they used to be. You’re in the throat of the death spiral now.
Let’s say you do some analysis and “discover” your mistake. You kill the campaigns that generate the $100 best customers and start generating $200 best customers again. How long will it take to get back to where you were?
Right. 6 months – at a minimum. Problem is, the campaigns that generate $200 customers are twice as expensive as the campaigns that generate $100 customers, and you’re now in the vortex of the death spiral. You spend like crazy but can’t make it up in time and at month 4, media costs outstrip cash flow and the company goes belly up.
Pareto plays a very tough game, eh? While you’re thinking about it, here again is a link to the Hagel post.