Or Behavioral Messaging, as we used to call it.Â
Much has been written about Measuring Engagement, but once you measure it, then what do you do with this information? Most folks know the idea driving the Engagement Movement is to make your messaging more Relevant, but how do you implement? Perhaps you can find the triggers with a behavioral measurement, but then what do you say?
This is the part Marketing folks typically get wrong on the execution side. They might have a nice behavioral segmentation, but then crush the value of that hard analytical work by sending a demographically-oriented message, often because that is really all they know how to do. So as an analyst, how to you raise this issue or effect change?
Marketing messaging can be a complex topic, but there are some baseline ideas you can use. Start here, then do what you do best – analyze the results, test, repeat.
You want to think of customers as being in different “states” or “stages” along an engagement continuum. For example:
- Engaged – highly positive on company, very willing to interact -Â Highest Potential Value
- Apathetic – don’t really care one way or the other, will interact when prompted – Medium Potential Value
- Detached – not really interested, don’t think they need product or service anymore – Lowest Potential Value
Please note that none of these states have anything to do with demographics - they are about emotions.  The messaging should relate to visitor / customer experience as expressed through behavior, not age and income.
These states are in flux and you can affect state by using the appropriate message based on the behavioral analysis. Customers generally all start out being Engaged (which is why a New Customer Kit works so well), then drop down through the stages. The rate of this drop generally depends on the product / service experience – the Customer LifeCycle.
Generically, this approach sets up what is known as “right message, to the right person, at the right time” or trigger-based messaging. Just think about your own experience interacting with different companies; for each company, you could probably select the state you are in right now!
OK, so for each state there is an appropriate message approach:
Engaged – Kiss Messaging: We think you are the best. Really. We’d like to do something special for you – give you higher levels of service, create a special club for you, thank you profusely with free gifts. Marketing Note: be creative, and avoid discounting to this group. Save the discounts for the next two stages.
Apathetic – Date Messaging: We’re not real clear where we stand with you, so we’re going to be exploratory, test different ideas and see where the relationship stands. Perhaps we can get you to be Engaged again? In terms of ROI, this group has the highest incremental potential. Example: this is where loyalty programs derive the most payback.
Detached – Bribe Messaging: You’re not really into this relationship, and we know that. So we are simply going to make very strong offers to you and try to get you to respond. A few of you might even become Engaged again.
Can you see how sending a generic message to all of these groups is sub-optimal? Can you see how sending an Engaged message to the Detached group would probably generate a belly laugh as opposed to a response? You’ve received this mis-messaged before stuff, right? You basically hate the company for screwing you and then they send you a lovey-dovey Kiss message. Makes you want to scream, you think, “Man, they are clueless!” and now you dislike the company even more.
Combine this messaging approach with a classic behavioral analysis, and you now have a strategy and tactic map. For example, you know the longer it has been since someone purchased, clicked, opened, visited etc, the less likely they are to engage in that activity again. Here’s the behavioral analysis with the messaging overlay:
Click image to enlarge…
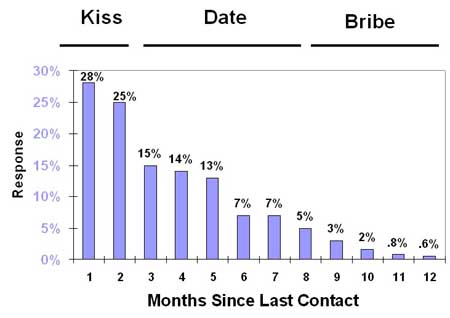
Please note “Months Since Last Contact” means the customer taking action and contacting you in some way (purchase, click) not the fact that you have tried to contact them!Â
So does this make sense? Those most likely to respond are messaged as Engaged – as is proper in terms of the relationship (left side of chart). As they become less likely to respond, you should change the tone of your communication to fit the relationship up to a point, where quite frankly you should take a clue from the eMetrics Summit and not message them any more at all (right side of chart).
Example Campaign for the Engaged: At HSN, I came up with the idea of creating some kind of “Holiday Ornament” we could send to Engaged customers. If the idea worked (meaning it generated incremental profit), we could do it as an annual thing; we could put the year on the ornament and create a “collectible” feel, which is the right idea for this audience. No discount – just a “Thank You” message “for one of our best customers” and “Here’s a gift for you”.
These snowflake ornaments were about $1.20 in the mail (laser cut card stock) and generated about $5 in 90-day incremental profit per household with the Engaged, test versus control.  Why?  Good ‘ol Surprise and Delight, I would bet.
We had some test cells running to see how far we could take this, and as expected, the profitability dropped off dramatically based on how Engaged the customer was. If the customer was even minimally dis-engaged – no purchase for over 120 days – there was very little effect.Â
Interactivity cuts both ways; it’s great when customers are Engaged, but once the relationship starts to degrade, folks can move on very quickly emotionally. That’s why it is so important to track this stuff - so you can predict when your audience is dis-engaging and do something about it.